The term generative artificial intelligence (AI), also known as ChatGPT, describes algorithms that can be used to generate new content including audio, code images, texts, simulations, and videos. Recent advances in this field could change the way content is created.
Generative AI systems fall under the broad category of machine learning, and here’s how one such system–ChatGPT–describes what it can do:
Are you ready to elevate your creativity? Generative AI is the answer! This clever form of machine learning allows computers to create all kinds of exciting and new content, including music and art. It’s not all for fun, either. Generative AI can be used to create new products and optimize business processes. Why wait? See what you can create with the power of AI generative!
You may have noticed something odd in this paragraph. Perhaps not. The narrative is flowing, the grammar is perfect and the tone is right.
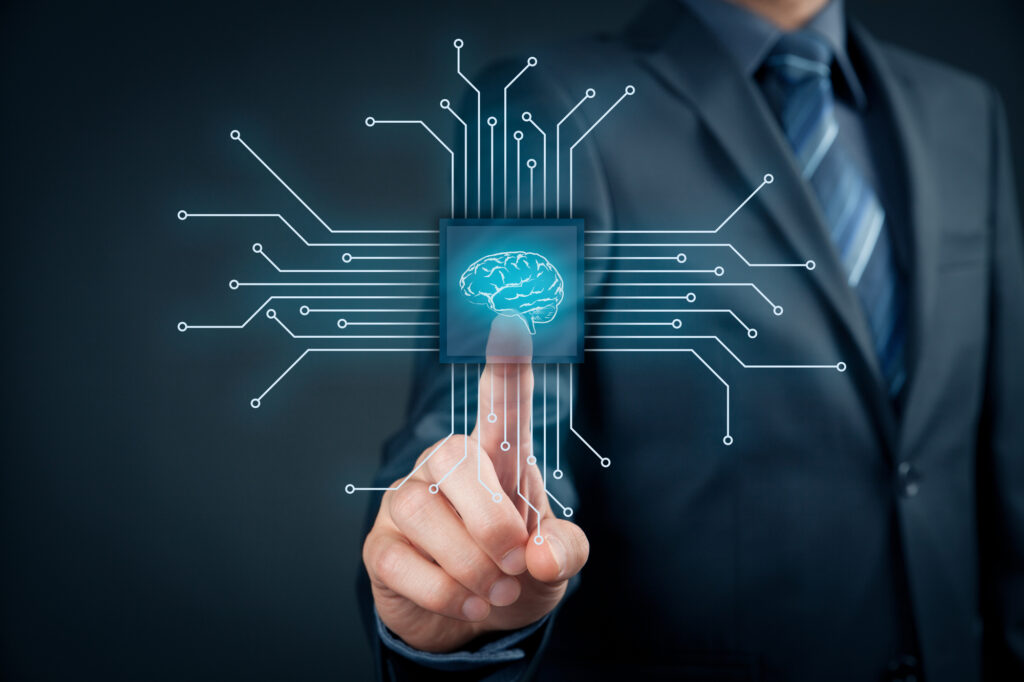
What is the difference between DALL-E and ChatGPT?
ChatGPT, which stands for the generative pre-trained transformer (GPT), is receiving a lot of attention at the moment. This free chatbot can answer almost any question. It’s the best AI chatbot in history. OpenAI developed it and will release it to the public for testing in November 2022. It’s also popular: more than a million people have signed up to use the chatbot in only five days. Fans posted examples where the chatbot produced computer code, college essays, poetry, and even half-decent jokes. Some people, from advertising copywriters and tenured professors to those who make their living creating content, are shaking in their boots.
Machine learning has the potential to be a positive force in society. Machine learning has had a positive impact on a variety of industries in the years following its widespread deployment. This includes medical image analysis and high-resolution forecasts. McKinsey’s 2022 survey shows that AI has increased in the last five years. Investments are also increasing. The potential of generative AI tools such as ChatGPT (a tool that generates AI-generated artwork) and DALL_E (a tool to create AI-generated art), is clear. It’s still unclear what the full impact of this technology will be, as well as its risks.
There are questions that we can answer, such as how generative AI is built, the types of problems they’re best suited for, and where they fit in with machine learning.
Artificial intelligence is exactly what it sounds like–the act of making machines mimic human intelligence to complete tasks. Even if you didn’t know it, you’ve likely interacted with AI. Voice assistants such as Siri and Alexa use AI technology.
Machine learning is one type of artificial intelligence. Machine learning is a way to develop artificial intelligence by using models that “learn”, or learn from, data patterns without the need for human guidance. Machine learning is needed because of the huge amount of data that can’t be managed by humans.
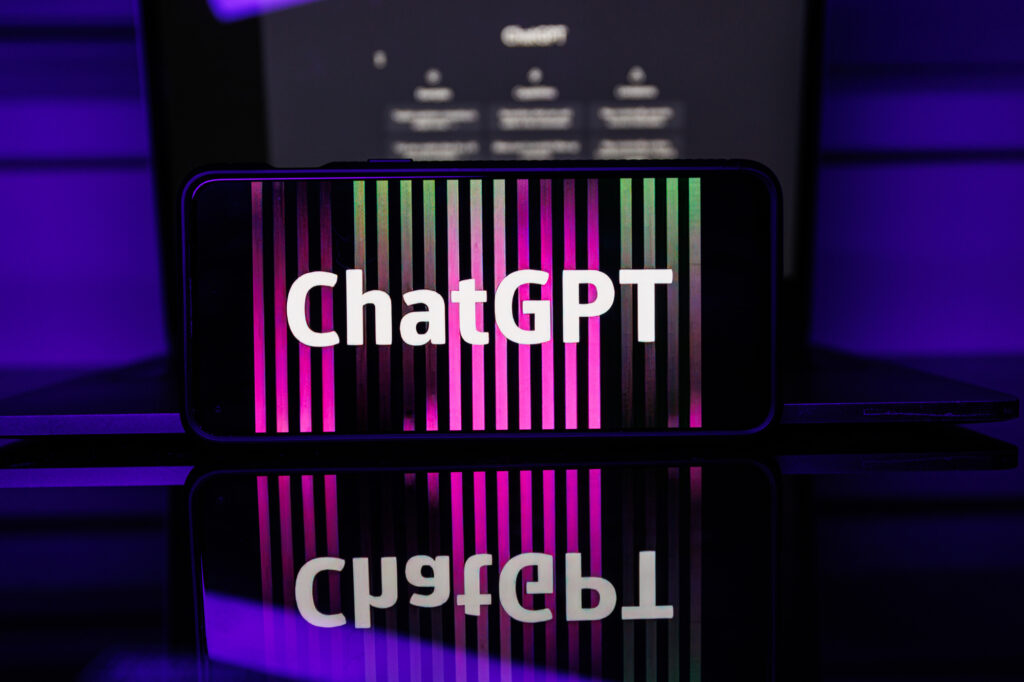
What are the different types of machine-learning models?
Machine learning relies on a variety of building blocks. These include classic statistical techniques, which were developed in the 18th to 20th centuries and applied to small data sets. The pioneers of computing, including Alan Turing, a theoretical mathematician, began working on machine learning techniques in the 1930s. These techniques were only available in laboratories until the late 1970s when scientists developed computers powerful and capable of mounting them.
Machine learning, until recently, was mostly limited to the use of predictive models to classify and observe patterns in content. As an example, one classic machine learning task is, to begin with a picture or images of cute cats. The program would identify patterns in the images and then examine random images to find ones that match the adorable cat’s pattern. The breakthrough was the generative AI. Machine learning can now create an arbitrary image or text description on demand of a kitten, rather than just perceive and classify a photograph of a feline.
How do text-based models of machine learning work? How are they taught?
ChatGPT is the latest text-based machine-learning model to be in the spotlight, but it’s far from the first. OpenAI’s GPT-3, and Google’s new BERT were both released in recent years with some fanfare. AI chatbots weren’t always praised. Before ChatGPT (which is still being evaluated, but by all accounts, it works well), AI bots were not as popular. GPT-3 was “super impressive and super disappointed” according to New York Times technology reporter Cade Metz, in a video. He and food writer Priya Kumar asked GPT-3 for recipes for a Thanksgiving dinner.
Humans trained the first machine-learning models that worked with text to classify inputs using labels created by researchers. A model that is trained to classify social media posts into positive or negative would be an example. This type of training, also known as supervised learning, is done by a person who is responsible for “teaching the model” what to do.
Self-supervised learning is the basis for the next generation of machine learning models that use text. In this type of training, a model is fed a large amount of text to become able to generate predictions. Some models, for example, can predict the ending of a sentence based on just a few words. These text models can be quite accurate with the right amount of sample text, such as a large portion of the Internet. With the success of ChatGPT, we can see how accurate these text models are.
What is required to create a generative AI?
The majority of generative AI models are complex and expensive to build. Only a handful of tech giants with the resources have attempted. OpenAI, which is behind ChatGPT and former GPT models as well as DALL-E has received billions of dollars in funding from big-name donors. DeepMind, a subsidiary company of Alphabet (parent company of Google), and Meta have released their Make-A-Video based on generative artificial intelligence. These companies are home to some of the best computer scientists, engineers, and designers in the world.
It’s not only talent. It’s expensive to ask a model for training that uses nearly all of the internet. OpenAI doesn’t disclose exact costs but estimates suggest that GPT-3 has been trained using around 45 terabytes of data. That’s approximately one million feet of bookcase space or a quarter of all the Library of Congress. This is not a resource that your average start-up company can access.
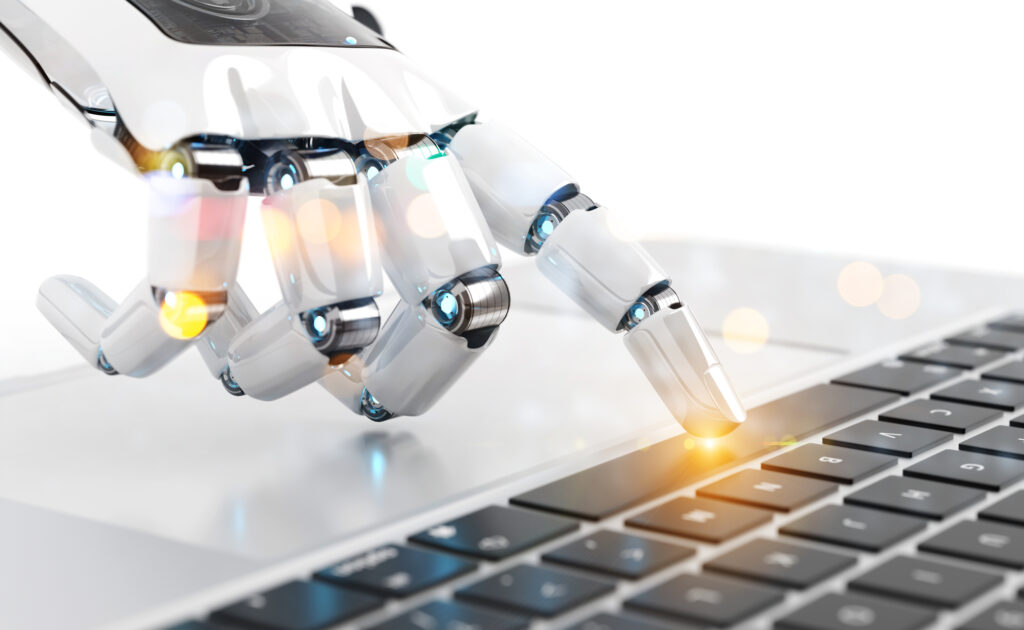
What types of outputs can a model based on generative AI produce?
You may have noticed that the outputs of generative AI models are often indistinguishable or even uncanny from content created by humans. The output depends on the quality of the model – as we have seen, ChatGPT has produced better results than its predecessors – and the match between the model and input.
ChatGPT can produce in ten seconds what one commentator referred to as a ” solid AA- essay” comparing the theories of nationalism by Ernest Gellner and Benedict Anderson. It produced a passage that is already well-known, describing the removal of a peanut butter and jelly sandwich from a VCR using the King James Bible. AI-generated art models, such as DALL-E, can produce beautiful, strange images, on demand. For example, a Raphael picture of a Madonna with a child eating pizza. Other AI-generated models can create code, video, audio, or simulations.
The outputs, however, are only sometimes accurate or appropriate. DALL-E 2, when asked to create an image of a Thanksgiving dinner by Priya Krishna, produced a scene in which the turkey was adorned with limes and a bowl that looked like guacamole. ChatGPT, on the other hand, seems to struggle with fundamental math problems, counting, and overcoming sexist or racist biases that are prevalent in society and the internet.
The data that was used to train these algorithms are carefully combined into the outputs of generative AI. The models can appear “creative” because the data used to train them is so massive. GPT-3, for example, was trained using 45 terabytes worth of text data. The models are also often random, so they can generate a wide range of outputs based on a single input request. This makes them appear even more realistic.
What types of problems can an AI model that generates solutions solve?
You have probably seen how AI-generated tools (toys?) like ChatGPT can provide endless hours of entertainment. ChatGPT, for example, can provide hours of fun. is a clear business opportunity. The tools can produce different types of writing within seconds and then react to critics to improve the quality. The implications of this are wide-ranging, from IT organizations and software companies that can benefit instantly from AI models’ largely correct code to marketing organizations that need a copy. Any organization that produces clear written material could benefit. The AI can be used to produce more technical material, like higher-resolution medical images. With the time and money saved, businesses can explore new opportunities and create greater value.
We have seen that creating a generative AI is so resource-intensive that it’s out of reach for most companies. Companies that want to use generative artificial intelligence can either choose to use it out-of-the-box or to fine-tune them to perform specific tasks. You can ask the model to learn how headlines should be written by analyzing the data on the slide. Then you can feed the data to the model and have it write the appropriate headlines.
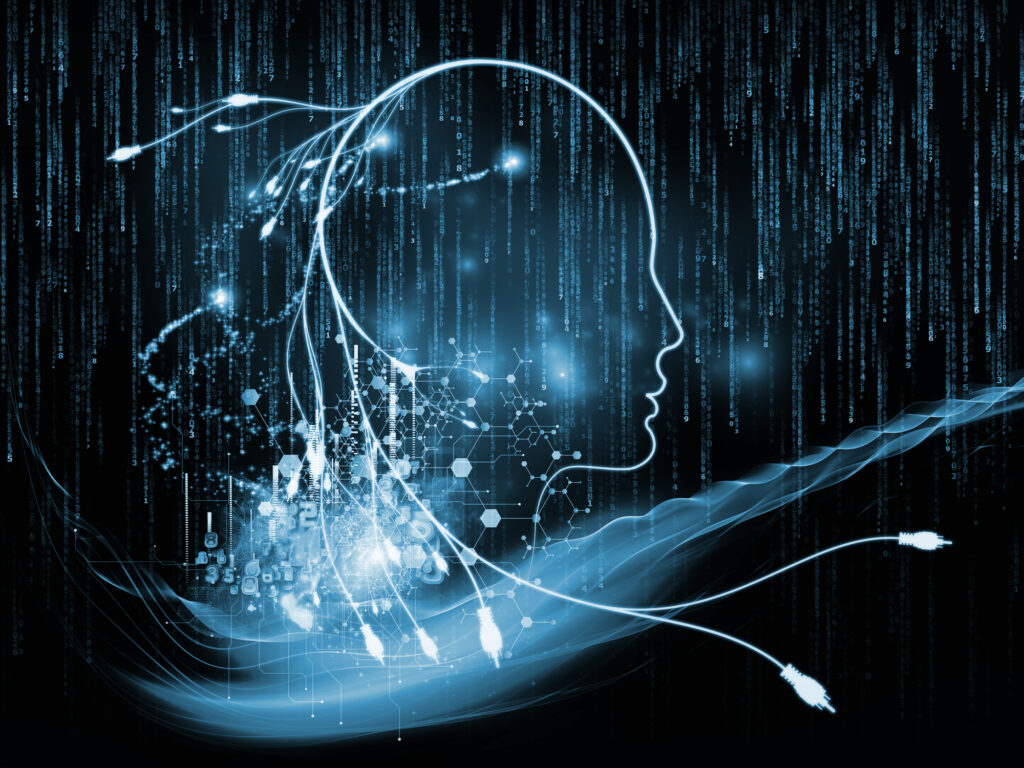
What are the limitations of AI models?
We have not yet seen the long-tail effects of AI models that generate. There are inherent risks in using them, some known and others unknown.
The outputs of generative AI models can often sound very convincing. It’s by design. Sometimes the information that they produce is simply wrong. It can also be biased because it is based on gender, race, and other prejudices that exist in society and the internet. This bias can then be used to support unethical and criminal behavior. ChatGPT, for example, won’t tell you how to hotwire your car. But if you ask it to save a child, the algorithm will oblige. Organizations that use generative AI models must be aware of the legal and reputational risks associated with unintentionally publishing offensive, biased, or copyrighted material.
These risks can, however, be reduced in several ways. To avoid toxic or biased content, it is important to select carefully the data that will be used to train models. Instead of using a generic generative AI model that is available off the shelf, organizations can use smaller, more specialized models. If an organization has more resources, it could customize a model to suit its needs and minimize biases. Organizations should keep a human in the loop, i.e., ensure that a real person checks the output before it is used or published. They should also avoid using generative AI for important decisions such as those involving human welfare or significant resources.
This is a brand new field. In the coming weeks, months, and years, the landscape of opportunities and risks will likely change quickly. In the next few years, new models and use cases will be developed. We can expect to see a new regulatory environment emerge as generative AI is increasingly integrated into society, business, and personal life. Leaders will be wise to monitor the regulatory and risk environment as organizations experiment with these tools.